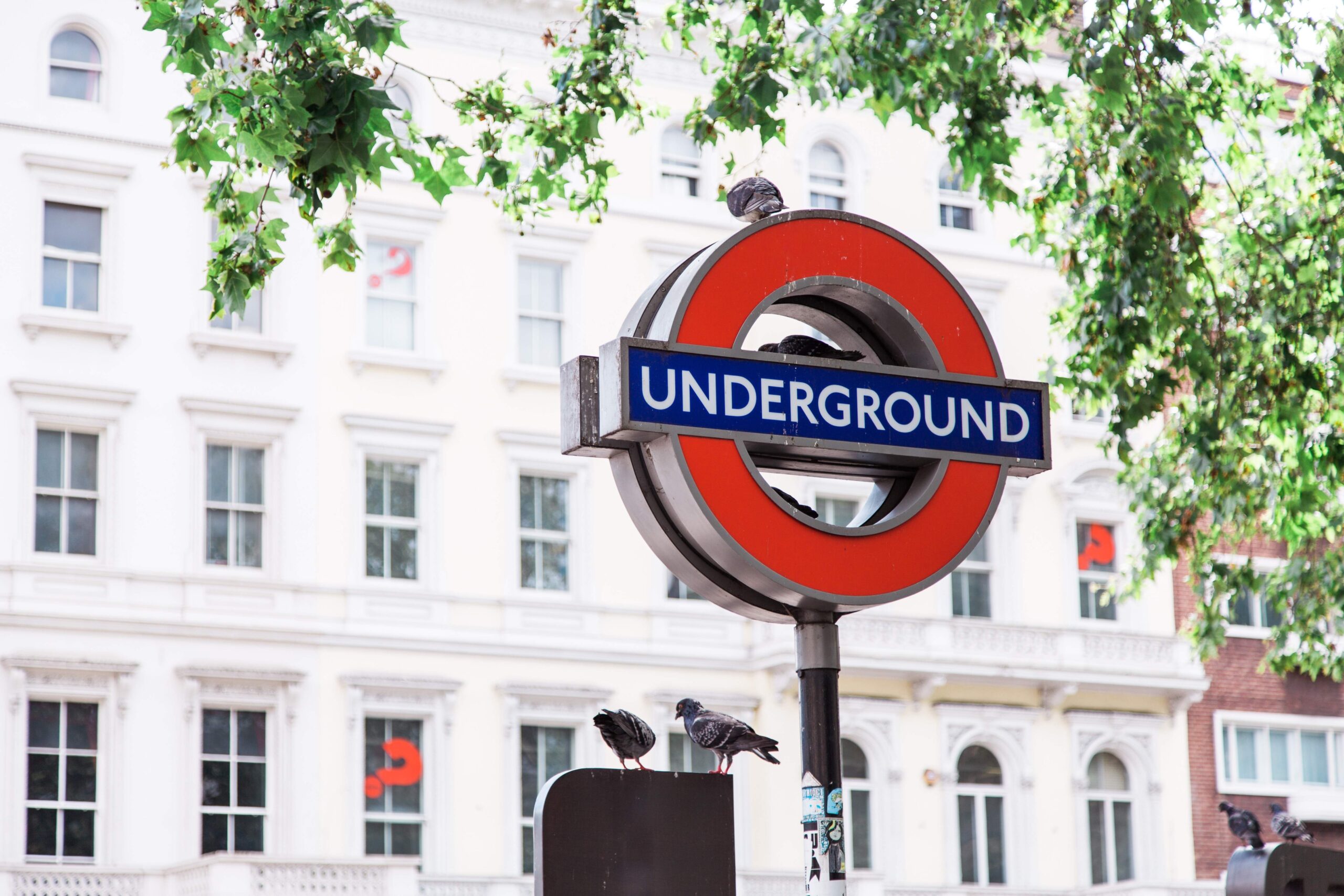
The London Underground welcomes more than one billion passengers each year and handles up to five million passenger journeys a day across its 500 trains.
Back in 2012, as smartphone consumption was rising among passengers – from 26 million smartphone users in the UK in 2012 to an expected 53 million by 2022 – Transport for London (TfL) started installing WiFi in stations across the tube network. Today, 97% of stations on the London Underground have WiFi installed.
These WiFi networks now collect and distribute a great deal of data, and with good data comes opportunity.
In the past, TfL has used depersonalised ticketing data, such as Oyster and contactless payment transactions, to analyse journey patterns and improve passengers’ experiences. Customer surveys, while not as reliable, provided additional information to help TfL understand passenger journeys better.
Through this research, TfL realised that the WiFi router network, which collects information about the connections made to the WiFi system, could be a far more cost-effective and powerful tool and, if successful, could eradicate the need altogether for labour-intensive customer surveys.
“On a typical weekday, we collect 19 million smartcard ticketing transactions that offer a valuable insight into the number of people using our network,” explains Lauren Sager Weinstein, the chief data officer at TfL. “But this doesn’t give us a complete picture, after they tap in, how do they travel? Depersonalised WiFi connection data can fill in the gaps, as it allows us to see how people travel beyond the gateline.”
How well do you really know your competitors?
Access the most comprehensive Company Profiles on the market, powered by GlobalData. Save hours of research. Gain competitive edge.
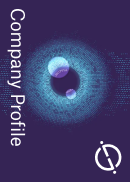
Thank you!
Your download email will arrive shortly
Not ready to buy yet? Download a free sample
We are confident about the unique quality of our Company Profiles. However, we want you to make the most beneficial decision for your business, so we offer a free sample that you can download by submitting the below form
By GlobalDataTo find out whether this could really be the answer to understanding passenger flow on the Tube, TfL ran a pilot in 54 stations from 21 November to 19 December 2016, to evaluate the usefulness of WiFi connection data.
Using data from passenger devices
In order to be completely transparent about the pilot, and to be clear that all the data being used would be 100% depersonalised, TfL worked with the Information Commissioner’s Office (ICO), which is the independent regulator of personal data in the UK.
Passengers were informed through marketing, focus groups and various methods of communication that the pilot was running and anyone who did not want to participate was advised to turn off their WiFi while travelling or to put the phone in airplane mode.
“Protecting the privacy and security of our customers’ data is of paramount importance and we recognise our responsibilities as a custodian of the personal data of millions of people,” says Sager Weinstein. “We understood that recording the location of a customer’s device MAC address at a specific place and time could be considered as personal data. We employed a number of controls to make sure the pilot fully complied with the Data Protection Act 1998.”
An encrypted, depersonalised version of the device MAC address – a unique number used to identify a device from others on a network – was collected. Along with the date and time, the device broadcast its MAC address, the access point it connected to – which would have been one of 1,070 – the device manufacturer and the device association type.
This data has great value to TfL for understanding passenger movement. “As we know where each access point is located – platform, ticket, hall – we can understand where in the station the device was when it connected,” says Sager Weinstein. “From the 54 stations included in the pilot, we collected 509 million probing requests from 5.6 million devices. King’s Cross St Pancras generated the most, with 37.6 million. The fewest were observed at Dollis Hill, where 10,000 were collected from seven access points.”
Big data techniques for analysis
Sager Weinstein says the team employed big data techniques to translate and interpret the information. “Firstly, we linked individual connections from a device so that we could create ‘journeys’ – an end-to-end trip that is comparable to but more detailed than what we get from our ticketing data,” she explains. “Using this approach, we constructed 42 million journeys from five million devices during the pilot.”
Further analysis created ‘movement types’, classified as: entry or exit, where device entered or exited network; pass-through, when a device passed through a station on a train; interchange, when device changed from one line to another; and sub-categories of movement, where a device was getting on or off a train.
The results provided a detailed view of how the tube network was being used in a way that had never been seen before.
Understanding the peak times, how busy the different line and route options are, how long transfers will take and how disruption will affect a passenger’s journey are all areas that can be analysed in real-time and used to help future passengers to streamline and improve their tube experience.
Understanding the busiest times
The WiFi data collected showed it was a successful way of quantifying crowding at a station at different times of the day. “Unlike ticketing data, which only shows customers entering and exiting stations, WiFi captures interchanges,” says Sager Weinstein. “This is especially important when measuring crowding levels in large stations where many customers change between services.”
While ticketing data can offer some insight, WiFi data adds detail, for example, it had illustrated the levels of crowding and demand at Oxford Circus station during weekday rush hour, and shows there’s a sharp increase in crowding between 8.30am and 9am, all of which is not possible with ticketing data.
“This data would be particularly useful to customers who would rather avoid crowding, even if it meant increasing their travel time,” she says. “It would allow them to re-time and/or re-route their journeys to avoid the busiest sections of the network.”
Identifying crowding at different line and route options
Being able to identify where and when crowding occurs means that TfL would be able to re-direct passengers in order to relieve overcrowded areas on relevant lines.
The results were gathered by calculating the number of people on the train based on where and when their devices connected to the WiFi network. “Traditionally, this information has only been available for 15 minute intervals and is collected when we survey our customers on non-disrupted days,” says Sager Weinstein. “WiFi data will allow us to provide continuous, responsive estimates of demand on specific services, enabling passengers to make informed decisions about the journeys they make.”
This could make a significant difference to journey times and the passengers’ experiences. For example, say a passenger used TfL’s journey planner for their route from, say, Camden Town to St James’s Park. Currently, they would be offered two options based solely on the estimated travel time and number of interchanges. Yet this route information would be vastly improved by employing WiFi data insight, as it could incorporate information that highlights busy areas and identify sections of the journey that are very overcrowded or have seats free.
WiFi connection data could also enable TfL to offer crowding information for individual trains. “If we can provide information for crowding levels on specific trains, customers may choose to wait for the next one if it means a more comfortable journey,” says Sager Weinstein. “This would also improve the service for everyone by smoothing demand over the peak period.”
Up to speed with transfers and disruption
Transfer times are another way that passengers can improve their journeys with WiFi data. The pilot, for example, showed how passengers move from the Northern line’s Bank branch northbound platform to the Victoria line southbound platform at Euston station.
While most take the shortest route, one-third take a longer route and around 5% take more complex paths. The times for each vary in length and this information could be useful for travellers who wish to have more detail about the time it will take to travel from station to station via the interchanges and avoid crowding in the station.
The fact that data can be used to inform passengers of the effects of events in real-time means they are empowered to avoid disruptions, where possible.
Overall, analysis of the results produced by the pilot has demonstrated to TfL that commuter WiFi data is crucial to understanding how passengers use the network and in providing information that will allow people to improve and take control of their tube journeys.
“Analytic tools and services built from this connection data can improve the way we run and plan our network, and can provide our customers with much more detailed information about their journeys. In view of this, we are now planning to roll out a network-wide collection process,” says Sager Weinstein.