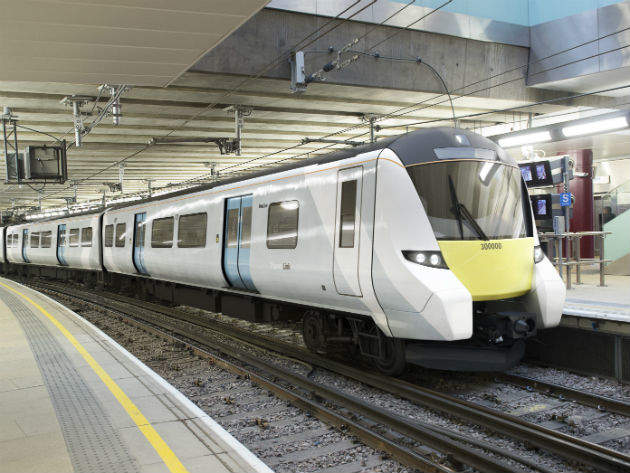
The shock posed by Donald Trump’s election as President of the United States showed the pollsters that big data analytics, when applied to the decision-making processes of erratic, emotional human beings, are likely to come up short. With even the most favourable polls giving him just a 1 in 3 chance of winning, the election has raised real questions about the limitations of data science and has damaged some big reputations in the process.
Nevertheless, when it comes to solid, reliable subjects like railway tracks, bridges and train wheels, data science is proving to be a highly effective tool, particularly when it comes to maintenance. By analysing thousands of data points on the condition of railway infrastructure, engineers are increasingly able to spot structural flaws at the earliest possible stage and intervene before they grow into more serious problems.
At the Institute of Railway Research at the University of Huddersfield, an increasing amount of time is spent finding new ways to collect and analyse this data. A project currently being carried out in partnership with Siemens, which came about after they won a remote condition monitoring competition launched by the Rail Safety Standards Board and Network Rail, should make this process much easier.
Void detection made easy
Normally, the UK rail network is surveyed by specialised track recording vehicles. These are in relatively short supply, so while major routes are regularly inspected, quieter ones are inspected much less often than they should be.
The project, known as Tracksure, involves the development of a cheap, easy-to-fit sensor that can turn any rail vehicle into a track monitor. The sensor is embedded in Siemens’ GSM-R cab radio systems and collects data about the condition of rails and rail beds by picking up track vibrations. Variations in these reveal the location of under-track voids, gaps between the sleeper and ballast that lead to an increased risk of rail breakage and generally result in reduced vehicle performance.
How well do you really know your competitors?
Access the most comprehensive Company Profiles on the market, powered by GlobalData. Save hours of research. Gain competitive edge.
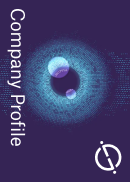
Thank you!
Your download email will arrive shortly
Not ready to buy yet? Download a free sample
We are confident about the unique quality of our Company Profiles. However, we want you to make the most beneficial decision for your business, so we offer a free sample that you can download by submitting the below form
By GlobalDataThe sensor transmits this information back to a ground system where it is analysed in depth by a team of data specialists who can decide whether or not pre-emptive maintenance is necessary. Using Tracksure, ground system analysts can apparently detect voids within a 5m margin of error.
Fixing a sensor to a normal train vehicle, rather than employing a specialised one, means these routes get the attention they need, and makes the process of track monitoring a lot less intrusive and disruptive. The network operator can make big maintenance cost savings and avert the need to close lines, re-route journeys and put in place speed restrictions. All this should ultimately lead to an increase in passenger comfort.
“This is one of a number of projects we’ve had with Siemens over the last five years focused on a range of things, but we’ve done a lot of work on maintenance optimisation,” explains Dr Adam Bevan, head of enterprise at the Institute. “Siemens have their in-cab systems, so the idea was to create a device that would supplement what was already in the cab. This is a neat way of achieving remote condition monitoring, including feeding data back to a ground system remotely, while using the communications systems you’ve already got in the cab.”
Project responsibilities were clearly delineated; Siemens handled the hardware and systems integration, while the Institute of Railway Research developed a new algorithm that could effectively detect the under-track voids and be programmed into the new sensor. Developing the algorithm took around 18 months, and Bevan is happy with the processing power of the final product.
“We started the project by doing some detailed data dynamic simulations, looking at it from a computer simulation aspect, modelling the track features and seeing how the acceleration signals within the train varied and what we could do with that data,” he explains. “From then onwards it was about coding an algorithm that would allow us to encode some signatures within a signal to indicate, in this case, latent track voids.”
Bigger data and machine learning possibilities
Recent on-train trials have been successful with repeatability, on different trains doing different journeys, found to be “excellent”, in Bevan’s words. With the void-detecting sensor now ready to go, the Institute and Siemens are looking at getting it on to as many trains as possible in order to get the broadest possible data sample. Tracksure will become a standard for Siemens’ cab radios in the next 2-3 years, as will a GPS-R/GPS antenna and GPS module to feed the data back to the ground system.
Siemens and the Institute of Railway Research are currently looking at how they can apply the same technological principles to other track characteristics, such as vehicle suspension faults and corrugation of the track or wheel flats.
Ultimately, the aim is to combine a wide range of data sources collected by as many trains as possible to create a kind of machine learning engine, a system of knowledge that ‘learns’ more and becomes more accurate with each interrogation.
“There’s a lot of research being done by ourselves and our academic partners about how you take this huge amount of data and turn it into something useful for both rolling stock and track engineers,” Bevans says. “This is both from a maintenance and engineering perspective as well as a rail systems, and operational one. The data from Tracksure is actually just one data set within that. You can imagine that you could combine it with data from other sources, such as a sensor on the track or maintenance data from Network Rail, and get a much better picture.”